BONUS: Building Your AI Organization
with Maia Brenner
October 5, 2021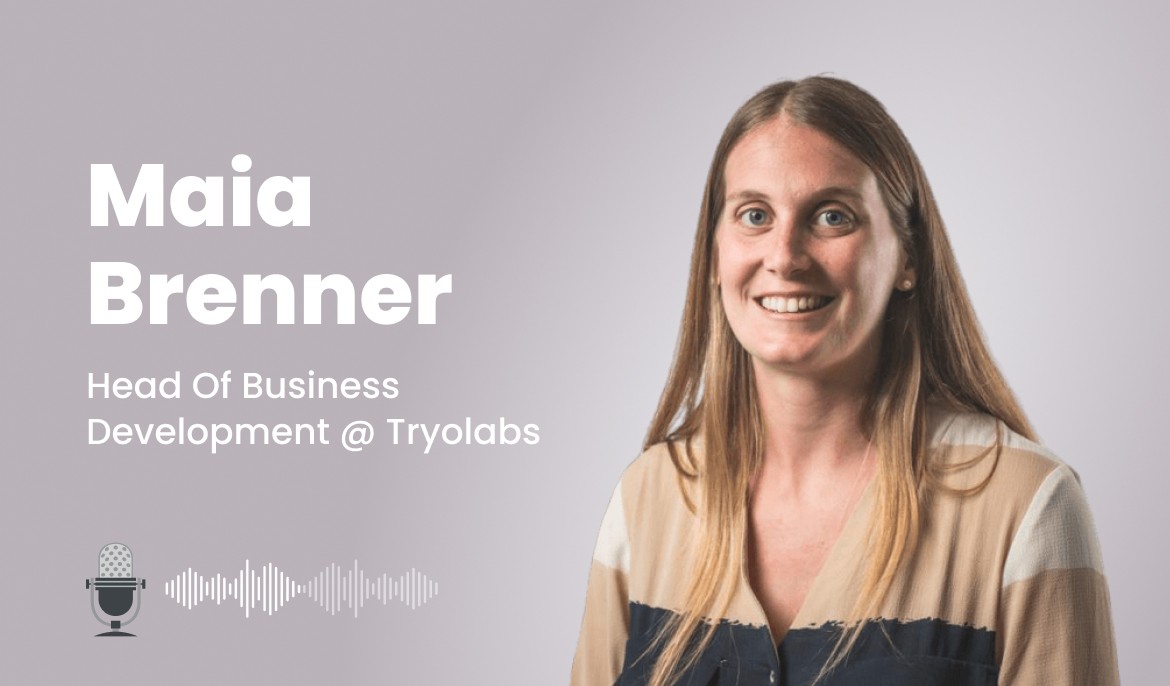
Maia Brenner, Head of Business Development @ Tryolabs
Maia Brenner is a passionate data scientist and economist with strong programming skills, a mathematical and statistical background, and work experience in consulting and the public sector. As an AI Specialist at Tryolabs she helps clients maximize the full potential of data science and machine learning to solve their business problems.
"Getting the alignment of the different stakeholders, the buy in and being able to measure and show the results... I think that's the biggest challenge that engineering leaders are facing right now with AI."
<cite>- Maia Brenner</cite>
Maia's experience in the consulting industry covers several projects related to demand forecasting, price optimization, customer segmentation, and natural language processing applications, among others. As a technical consultant, she has helped design and develop AI solutions for companies from several different industries such as Retail, Finance, Pharma, Logistics, Transportation, Hospitality, Education, and more.
She is also a professor in several universities and enjoys working on initiatives of AI4SocialGood. She has helped in the application of Machine Learning to improve the Public Education sector and is involved in Gender Inequality research groups.
Show Notes
- The origin story behind Tryolabs (2:33)
- Common AI/ML challenges Tryolabs helps solve (5:48)
- Most painful problems with building AI capabilities (7:50)
- What are the fundamentals to build an AI organization? (10:11)
- How do you integrate AI/ML into your core business? (12:42)
- What problems can (or can’t) be solved with AI/ML? (15:18)
- How Tryolabs helps companies to identify specific AI/ML use cases (16:59)
- Common pitfalls when investing in & integrating AI/ML into your company (18:19)
- How to start small & experiment with AI/ML solutions (20:14)
- How Tryolabs scopes & iterates their AI/ML projects (24:42)
- Metrics, KPIs & other ways to determine feasibility, impact & ROI of your AI/ML project (26:53)
- How to build an AI/ML roadmap for your organization (30:34)
- How Tryolabs accelerates building your AI organization (34:28)
Ready to own your AI Strategy? Learn more about Tryolabs HERE
Transcript
The origin story behind Tryolabs
Patrick: I think just to speak to the person listening into this conversation, the thing that's going in their head is probably something along the lines of this... "I know I need to invest in AI and I'm probably getting a ton of pressure from the C-level executives to figure this thing out. But I have no idea where to begin. Do I start with a data scientist? Do I bring in a platform or service? What do I do? And where do I begin?"
And so Maia welcome to the engineering leadership podcast. Thank you for being here to help us answer this question for the members of our community.
Maia: Thank you very much for the invitation. It's a pleasure for me to be here.
Patrick: Absolutely. So I think before we get into the topic, Maia, can we talk a little bit more about Tryolabs first and just get introduced to the company and what you do?
And so can you give us a little more about the origin story of why did Tryolabs first get started and how did you all get involved in the AI ML space?
Maia: Yeah. Sure. So Tryolabs started as a crazy idea from 3 friends, three Uruguayan friends, Ernesto, Martín and Raúl who were thinking on not starting AI machine learning consulting firm as it is today, but they were definitely thinking that AI would be a great impact to several different businesses. And they knew how to apply some kind of AI and Python to different problems.
So they started thinking, okay, how can we start working on this and how we can help other companies make use of this new technology. So this started more than 10 years ago and there is like a internal discussion, whether it was our 11th birthday this year or 12th birthday, because they started in a garage.
And so when is the official start date of the company? It's not very clear, but anyway, it was long ago. So, it's even crazy to think that by that time, when they started Tryolabs, there was no Kagel machine learning competitions. The ImageNet dataset was still not released. There were many things in, in the AI phase that we know nowadays as the normal thing that we talk about that were still not there.
So I think they had a great vision and they started working with Silicon Valley early adopters, mostly startups from Uruguay serving those companies. But right now we have more than 60 people working from Uruguay. We also have offices in Luxembourg and San Francisco. Most of the clients still are in the U S but we're seeing a lot of adoption from other regions as AI is getting everywhere.
So from the European market, from the Asia market, we're also seeing a lot of adoption. And thankfully right now from Latin America and South America itself, we're also seeing that there's great interest into getting into becoming a AI organization.
Patrick: I'm a little bit like, wow, it's been 10 years since the organization has been around. It's like, this is really early, early AI, like very early adopter trend.
Common AI/ML challenges Tryolabs helps solve
Patrick: So you mentioned Tryolabs is about AI consulting and helping people learn how to leverage AI as a technology within their businesses. Can you speak a little bit more to the problem that Tryolabs typically helps companies solve? What's the focus of Tryolabs? Like what are some of the problems that you all have supported different companies with?
Maia: Yeah, I think that's a really good question because I think there's different problems that we help to tackle with AI, but also different stages in which companies out and that we started engaging. So some of them are in the really early steps into defining what's the best AI strategy and the roadmap.
So we help them into identifying those potential use cases and diving into those ones. And others already come with a very specific idea of where they already know they can apply AI. So we help them in their development of those solutions. So we like to call ourselves, like end to end AI developers, because from the very beginning of defining the idea or the roadmap of the project as to the very end of developing, and making sure that that project and that development that we did is put into production and that we are there to support in the whole process.
So that's like in the different stages and in terms of like, the verticals as we call them, we have like three main areas of expertise that are within the AI spectrum that's everything that has to do with computer vision solutions that is understanding and analyzing video and images, then everything that has to do with natural language processing.
So understanding text and audio too. And then what we call predictive analytics solutions, that's everything that has to do with forecasting tabular data. So there are applications such as price optimization, demand forecasting, supply chain optimization solutions that we build within that vertical
Most painful problems with building AI capabilities
Patrick: That's great. Thank you. I think you were sharing a bit about the history of Tryolabs. So you all have seen a lot of things in the space, a lot of challenges and have supported people through a lot of difficult applications of the technology. Can you share a little bit about, like, from your perspective, what are some of the most painful problems that you're seeing engineering leaders face when it comes to building AI capabilities?
What are some of the high friction areas that are really challenging for people right now?
Maia: I think as you name it at very beginning, there's like a really big push as to develop AI, but people don't really know how to do it. And it for engineers leaders nowadays difficult to get their buy in from several different stakeholders as to be able to invest in that and measure and show the results of what you're doing.
So everyone is saying, okay, we should be investing in AI. But then when it comes to the moment in which we have to define, which is the project, how many people do we have to put in the project, the resources, the investment we need to have. It's like, I'm not sure because I'm not sure what I'm going to get from that.
So in general engineering leaders or other areas in terms of engineering it's very certain the output you will get from the investment you are doing, but when it comes to AI, there's like a very big uncertainty of what's the result and the outcome you may get, because it will definitely depend on the data that you have and the skills.
Patrick: And even on the problem, the solution you are building, you may not have like the exact outcome you will get. So getting the alignment of the different stakeholders the buy in and being able to measure and show the results. I think that's the biggest challenge that engineering leaders are facing right now with AI.Thank you. To summarize the challenge here, because I think you really captured some of just like the dynamics at play that make this a really difficult thing. One is first off, people just don't know how to go through the development process. The second is that like the alignment and the buy-in that the stakeholders you're working with may not know the value. And then the third being that the uncertainty of the result that you produce may or may not yield the intended outcome that you want to start with. That is a really difficult environment to operate in! Like I can see why that is a really painful challenge for people to navigate.
What are the fundamentals to build an AI organization?
Patrick: And so I think specifically speaking for the first one of like a lot of people wants to develop, but may not know the clear pathway to do it.
I was wondering if we could start and dive into the fundamentals here of where people should begin with this. So can you share a little bit more about when you all are getting ready to, or consulting and working with other organizations to start their AI project or to build out an AI capability? What are the fundamentals here to building out your AI organization?
Maia: So, first of all, I think the most important part of it is to demystify AI. We need to understand what are we talking about AI and understand that it's not a silver bullet that will from day one, erase all the problems you have and magically solving everything. So that's not what artificial intelligence will do, but we have to de-mystify and get everyone else to understand what are the kinds of problems that AI can, and which ones cannot be solved with AI.
AI And I think apart from demystifying is, as I said, like getting the buy-in and getting everyone to be aligned on what AI can get us a results and build the confidence and the board, all the workers at every level, because there's really this idea in everyone's head that AI is here to replace us the humans, and that AI will probably end up with our jobs and I think it's very important as to start building that confidence that AI is not here as to replace us, , or to diminish our work, but it's going to be able to enhance what we are doing as maybe automatizing some very repetitive work or some tasks that do not need much of human creativity, but there's a lot of things that definitely where humans will definitely be giving a lot of values when having to crack the problem, define what's the problem and define the solutions and being able to, as I said, use creativity and use our imagination as to solve those problems. So maybe more repetitive tasks that do not need much of that creativity will be able to be automatized.
And so we will be able to all take more value of our work. So I would say starting by building the confidence at all entry, all company levels, that's like the first thing to be doing in their organization if you want to become an AI organization.
How do you integrate AI/ML into your core business?
You mentioned integrating into the core of the business and helping people understand, like how do you actually integrate AI into the core of their business?
Patrick: Is there an example or maybe like a, like a common misconception where the business wants AI to do X, but it's not integrated into their business... Can you help us better understand? What does it look like to actually have that conversation where you're integrating AI or ML into the core business?
Because I feel like that may be a trap for like a strategic partner to be like, "Okay, we want all the benefits of the solution, but we don't actually want to do the work to integrate it into the core product." Can you help us understand a little bit about the distinction or what that looks like?
Maia: Yeah. Maybe like one example that comes to my mind, a project I'd been working before shifting to this new role as a machine learning engineer. It was on, on a price optimization project. And so when I think a lot of people are thinking, okay, we should be using AI for doing dynamic pricing, price optimization.
I don't know. We know Amazon is changing prices very, very often. So we want to do that. And then I've seen many times like business people do not realize how sensible it is to be changing the price of what you're selling. So they are like willing to do AI and do something like magical in terms of having something being changed more often.
But it's not that they really want to change their pricing strategy. So at the very end, they may say, okay, we're just following competition. And it's like, okay, but we are not optimizing the solution. We are just like doing it faster than what you're manually doing, but it's not that it's getting to the core of your business.
And so in order to get an optimal pricing solution, you'll probably need to be aligned with the supply chain and logistics team as to understand how much inventory do you have in your stock. You have to work with the marketing team as to define what are the promotions that are being run in each moment in time. You need to work with the business and the market analysts to understand what's going on in the market. And you need to align with a lot of pieces. It's not only like plug and play with a new software as to see how price changes often. But to have like everyone align into that, that's going to be very core to your business.
Patrick: That's an incredible example because I think it's not just about changing prices, but it's changing all of the other functions in the organization to leverage the strategic benefits that happen when you do that. That's a great example. Thank you.
What problems can (or can't) be solved with AI/ML?
Patrick: The other thing I was curious about, because like you're coaching people around, like the problems that can, and can't be solved all the time early at communicating, like what can and can't be done.
Do you have any tips or things that you do to educate people around what can, and can't be solved with AI or ML? Is there anything that comes to mind or tips or strategies that you could share with us there?
Maia: Yeah, I think for instance, when you think about computer vision tasks or natural language tasks, It's all about like something that the computer, or you may be looking at an image or maybe looking at a certain text. And so if there's something that just by looking or just by pressing about ourselves, you can do very, very fast.
And you can understand from that, having a look what's the output you have to do. That's probably something that can be automatized. So we like to say, like, if there's something very, a task that is very repetitive that you can do in five seconds. So probably that could be automatized with AI. So for instance, if you're having a look at an image and you need to retouch like the image as to take out the background, and that takes you like five seconds as to do, or maybe 10 seconds, an AI algorithm will probably be able to do it in less than 10 seconds and you won't need that. Or for instance, if you need to have a look at a new clothing to see, is it authentic or not? It's not authentic. And you just have a look at it and you understand it probably that's something that AI can do. Or the simplest example, it's like, okay, I get to see a cat and a dog and I can perfectly well identify which one is a cat and which one is a dog that's kind of the things that AI can definitely do.
How Tryolabs helps companies to identify specific AI/ML use cases
Patrick: So when you're working with different companies to help them define their use cases, are you sitting down with them and sort of going through like, okay, these specific tasks that your team are working on are happening under five seconds. So we could probably figure out a way to automate this with AIML. Is that what that conversation looks like when you're working with different partners?
Maia: Yeah, definitely. So when we start on this, like AI roadmap kind of projects or AI strategy project, one of the most important parts is to understand like the business and the main pain points. So we organize different focus groups or different interviews with different company groups and teams as to understand what are the kinds of tasks they are doing and which ones of those they identify as the pain points. So from there we can take ideas and we started them brainstorming on what kind of solutions we could be working.
I'm very much into a design thinking approach as to think of solutions integrated with the business experts and doing everything like side by side, because at the end of the day, the people that are working on the business on the, on the day-to-day are the ones that better understand what, and what cannot be done once we explained like what AI can do and cannot do. So those are our champions that identify those use cases.
Common pitfalls when investing in & integrating AI/ML into your company
Patrick: You mentioned roadmapping. I definitely want to dig into that a little bit further, but before then you helped us understand kind of the core fundamentals of building an AI organization. And I think you'd mentioned that it is not a plug and play technology, and I feel like that's a misconception. That's a pitfall that somebody could have.
I know for me, I'm like, oh, like it's something you can just like, it's a pretty box. You put it into the thing and it works. Are there other pitfalls that people typically bring to this conversation about integrating AI into their organization that you could call out for us?
Maia: Yeah, I think one of the main pitfalls that people in general end up getting into it's thinking that more data is always going to be better, so, okay. We don't have enough data. So the issue here is that we need to get more, more and more data. And so we may go and buy different data sources and such. And I think that's like a really, really bad misconception because the data needs to have certain quality because in AI and in all the data science applications, we know that garbage in, it's going to be garbage out.
So if your data and the more data that you gather, it's not that good quality, and it's not representative of what you want to do then. So probably having more will only make it either more difficult because , you will probably take more time as to analyze that data and understand how bad it is.
So in general, I think like the common pitfall is like thinking of a really, really big project or really big application with a lot of, lot of data and getting into big data platforms and such. And I think many times its way better as to start with something small and be able to show and measure the results with that small problem, small project that it's at the end of the day very valuable.
How to start small & experiment with AI/ML solutions
Patrick: When I imagine building out an AI capability... I imagine a huge long time horizon project that requires a huge investment.
How do you break that down and start small? Like, do you have an example of maybe what that looks like to take this big AI capability project and break it down to one small experiment to do that can help showcase the business impact?
Maia: Yeah, certainly. So one of the project I mentioned before that was in terms of price optimization was from one of the largest travel retailer company in the world. They have more than 100 stores and they have hundreds of thousand SKU as to optimize. And they knew they could get a benefit from making their pricing strategy more data driven and optimizing the pricing strategy.
They had that gut feeling. They weren't sure like how much to what extent that AI solution could help them leverage. So they were expecting like an increase in 1% in gross margin and they would be happy with that.
We started and say, "Okay, we know there's a lot of room for improvement here and there thousands, a hundred of SKUs we can start working with, but we need to get the right mindset and the experimental approaches to make sure that we're going in the right direction.
So we started defining a POC, so a proof of concept project that was only taking into consideration 150 SKUS So it was pretty small in terms of the number of SKUs but it was very much designed in an experimental way as to be able to measure and show the results that that experiment would have.
So. We had two different stores. So one we use it as a control store and the other one as a treatment store. And they were in different airport so it was in terms of experimental design, the ideal because they were completely independent. People couldn't move from one to the other, knowing that prices were lowering one from the other.
And so we started with that experiment. We optimize 150 SKUs, we did all the system as to be scalable for more SKUs. And in that particular case, we were able to showcase that the price optimization solution with AI increased the gross margin by 28%. So they were expecting a 1% increase because they had the gut feeling.
But we could show them that there was pretty a lot of room for improvement. And so I think having that experiment and mindset at the beginning was like the way to start building that capability.
And of course, if you see it was small, but the system and their approach and everything that we were developing was able to scale to thousands of hundred SKUs and that's what they are doing right now.
So I think that's kind of how you can think of that big, big problem getting into something way smaller.
Patrick: I mean, the results from that are staggering.
Maia: Yeah, in terms of how long that would take. So you would think, "Okay... optimize the unchanging, all prices for everything and integrating this system with a hundred thousand of stores I have in several different continents and such would take like ages to perform."
So the POC t ook almost three months, and then obviously you will have time to integrate that to different systems, some different stores and such. But being able to have like a result and have everyone convinced by three months that this is working it's way better than having to wait for two years as to see whether it has worked or not.
Patrick: I think that's such a great point for the three months. And I'm so blown away, like a 28X result. They had an expectation of 1% improvement and you were able to deliver 28% improvement? Like that to me would be a jaw-dropping if I'm reading that results of like, should I invest in this? Like, that seems like it'd be a pretty loud yes...?
Maia: One point of that, it's like, we were also surprised, right? Because as I said, like, there's a lot of uncertainty when you get into this project so we cannot promise anyone will get a 28% increase in gross margin because all processes, all strategies and all data will be pretty much different in all cases. So we were also even surprised by this.
And then at the end of they really doesn't matter whether it was 25 or 29 or something around it. There's room for improvement and we are going into that direction of improving. this is gonna work, If you're not applying any data driven strategy or machine learning AI , capabilities there probably is room for improvement with this technology.
How Tryolabs scopes & iterates their AI/ML projects
Patrick: Definitely!
How much iteration happens to the model after that first experiments results? Like, are you dramatically changing or tweaking or adjusting it? if you achieve 28 x, the result that you're expecting, what happens after that?
Maia: So in general, how we scope project, it has like three phases. So the first one would be the business understanding and data understanding clean preparation. So everything that has to, to do with getting your data ready.
Then the machine learning and development. So there you'll have a lot of different experiments and different things to test out and things. It's not that you have one model, then you know that it's going to be working. But you have to try out with the data that you have, what other feature, what other data that you can integrate. And so you're already experimenting and trying out different things within that phase.
And then what we call the experimental phase in which we are testing it out in there in the field. Right. And so even when you're testing it out on the field, you're still iterating to improving the model. And I think it's very much related to the business and the marginal improvements you may get.
So if you ask a machine learning engineer, they will probably always tell you they need another sprint as to improve the model. And there's a lot of things that could be improved and that are not perfect. But many times the marginal increase of that improvement will not move the needle. So at some point you need to say, "Okay, this is good enough."
and probably you'll have to retrain the model with new data, with the latest trends on such. But maybe not investing in really fine tuning that model into the most detailed thing, because you may not ever end up improving that, right.
And so I think it's being able to balance the trade-off of "Okay. What's the investment you're going to be doing into improving on what's the return you may get from that improvement."
Metrics, KPIs & other ways to determine feasibility, impact & ROI of your AI/ML project
Patrick: Maia, we've been talking a lot about like how to help educate the company on AI use cases, ways that you've measured impact in the past, and designed experiments around like the price optimization here.
Are there other considerations that you have for determining the feasibility, impact, And ROI of different AI initiatives? Like, are there other elements for your framework for how you would approach scoping out that different initiatives?
Maia: Yeah I think on what we were talking before of being able to measure the marginal impact or increase that investment will have. It has always to be related to the business metrics. Right?
So imagine that we are improving, as I said, in 1%, this solution into gross margin. We are always translating the technical metric into a business metric that has to make sense in terms of the business.
So if we are reducing costs, the solution shouldn't be aiming us to be the most precise, but to be able to impacting in the business metrics. So I think making that translation is very important as to define which one is the use cases that you should be investing.
So you need to analyze those in terms of the technical feasibility, how difficult it is, and what's going to be the business impact that having that solution will provide you with
Patrick: That definitely makes sense.
I had a question about metrics and measuring some of the KPIs. So you talk about measuring the business impact, and then I have to imagine, like there's some sort of measurement around whether or not the AIML model that you're using is working as well.
Are there any KPIs that you found to be effective for AI goals for an organization?
Maia: Yeah. So there is some, for example thing out of our bridging, we were working last week in which there were like three different models in terms of image classification and object detection that we were testing out. And there was like a huge discussion whether we should be optimizing for precision or recall in terms of what's worse in terms of customers engagement. If we have a lot of false positive is instead of not having the false negatives.
And so it was very technical in terms of, of what was the discussion about. But has a lot to do with how people will interact with a solution on what's going to be the, the engagement they will have. But then another metric that they came into, I was like the throughput. So how long it will take for a certain model to be performing or doing inference in production.
And so those are kind of the things that we always need to consider and to be aligned the business expert. Because maybe the best model that we are trying out at the best solution that we have from the technical point of view, it's A but then when we use it in with our clients and customers, they are expecting to see B in terms of, I don't know, the time it will take to run this certain outcome or the kind of explainability tools they want to see when, when they're interacting with these models.
So I think it's always important to have in the table, people from the business side. If you're designing our products or product managers that know how a customers will use your final product with a technical team, because if you're only pursuing technical metrics and so machine learning optimization metrics, you'll probably be blinded into what will be needed in terms of the client or final customer.
So, KPIs, from my perspective, should always be set from the business side and aligned with the technical team.
How to build an AI/ML roadmap for your organization
Patrick: Can we talk about roadmaps and how to start to build a roadmap, to help people build out their AI organization? Where do you start and how do you approach building a roadmap?
Maia: So, to start thinking of the roadmap, we have to get a very good understanding of, of the business and what are the pain points? So starting by having a look around us, like, what are the processes? What are the pain points? What are things people or tasks that people are doing within your organization that you think could be done in a better way if we're using more data driven approach?
So that may be having focus groups, interviews, or just watching people day-to-day work. That's like the first step. I would say that probably will take like three or four weeks as to be able to understand what's going on. It really depends on the size of the company, how many different teams you have working with.
And then assessing once you have the identified those pain points and what could be potential use cases for AI, you need to assess the feasibility. And as we said, not only the feasibility, so is this possible to be done or not, but also to see what's going to be the impact.
So if we are able to solve this and say that we automatize this process that takes three people, 20 hours per day, we multiply it by X amount of days in the year... so what's the business impact this solution will have? Looking for that project or that use case that will provide impact and that it's feasible to be done. It's I think the step to go as not to engage and commit to a very, very big project, that it's going to be very difficult to put in production and also to show the results it has.
Then once you have identified that lowest hanging fruit, studying, building a POC to prove the concept and to see whether what you have thought could be a good solution... is indeed, a good solution. So starting with that end to end baseline model thing, it's the best thing to go at.
As I said, if, if you ask a machine learning engineer, they proudly say this will take months to develop. But we did just like the baseline approach. And then we'll have time to continue iterating to have improvements on that. But probably that would take like also couple of weeks as to have like the end to end baseline solution. And then continue to iterate and test and learn. Right.
So once you have that baseline model, you test it out with a bunch of products or clients and you see how it's going and you perform some experiments that approach us to measure whether it's better or worse for what you have been doing so far. And then continue to iterate and learn from that experience.
And if you already have proved the concept for that one, you will probably be able to grab another project. I'm started these like iterative cycle as to start again with a POC and start again with the experimental approach, showing that it has an impact and they continue to improve and build on.
Patrick: I'm listening to you sharing the approach to how to build the road map and I'm like, "Man, that sounds so easy."
But I have to imagine, like in the details of all of that is where the challenges come into play. When it comes to identifying, where do you even start? Can we even do this? And what experiments do we do to prove the concept?
Maia: Yeah, I think maybe one thing that I forgot to mention is being able to have people ongoing that process several many times will allow you to continue to grow that muscle, the internal capabilities.
And people will already have takeaways, learnings and out of retros of what has worked and what has not worked that fine. And that's the way in which you will be able to continue to grow your internal capabilities
How Tryolabs accelerates building your AI organization
Patrick: Absolutely. So Maia you've walked us through the fundamentals and the pitfalls, and even now just sharing on how to build a roadmap. And I think at the beginning of. What trio does is support people through this whole end to end process. Whether, you know, people are just starting and have no idea how they want to integrate AI into their organization, all the way to helping people who have a specific use case and want to develop a solution. And so you all are really experienced with being able to help people through all different phases of this process.
Can you give us a little bit more insight into what does that look like to bring in a partner like Tryo, to be this sort of accelerating force within the organization?
Maia: I think bringing in a partner like Tryo,is like, as you said, accelerating, but also growing your internal muscle because we are working always side by side with our client. It's not that we are like separated team in your organization but we are there side by side with it... even though we are right now remote, but we can feel that closeness to our clients as to be able to understand the business. We sharing our experience and knowledge on how we have solved these kind of problems on the technology we use on our end.
So we not only help in the acceleration of developing the use cases, but also in the process of building those capabilities internally. So in some cases we even help hire data science leaders if they haven't done that before.
And in cases in which they already have data scientists or data science leaders and teams, we're always working side by side as to share our experience and our knowledge as to all be able to grow together into being better AI professionals in the field.
And that's part of what you can expect of engaging with Tryolabs is learning about great good practices in AI and being able to test out very fast, the ideas that you may have in house. And then being able to continue to develop many other use cases, because as we always say, like, there's not going to be a stone unturned that will not get touched by AI, and it's going to be probably in all industries and in all business... probably everywhere.
Patrick: And if somebody wanted to learn more about tryout or to stay in touch, What would you recommend people do? Is there a website you want to point people to? Or how can people follow up and stay in touch with.
Maia: Yeah, certainly. So we have our websites, tri labs.com. We also have a blog post in which we'll always share like these kinds of experiences. And it's, in some cases it's pretty technical, but I encourage people to dive into the blog versus
and we also have our email: [email protected] in which you can reach out to us or even you can reach out to me. It's pretty easy, my email is [email protected]
So very open as to discuss, even if you don't have the particular use case in mind... you want to hear more about what's going on in the industry? What other players are doing regarding AI on the business industry you're working with, we are very happy to connect and we also continue to learn every day from your clients.
I got some resolve of the problems and challenges they are facing.
So we are very open and glad to stay in touch and be connected to learn about.
Patrick: Thank you, Maia. And I've certainly learned a lot from murals blog post. Everything from developing AI capabilities to the case studies that you all share are really, really fascinating. So definitely great things to check.
Maia, I just want to say thank you again for an incredible conversation and helping guide us through what can be a challenging and confusing and difficult journey to build an organization with a challenging technology like artificial intelligence, like to build that out for the first time is a big hurdle. So thank you so much for being a guide for our community to help people understand how to approach this tricky problem. So, Mike, thank you so.
Maia: Thank you very much the opportunity to share our experience.